🤖Reinforcement Learning
🪞Imitation Learning
🛡️Alignment and Safety
🧠Representation Learning

Fast Adaptation with Behavioral Foundation Models
Harshit Sikchi,
Andrea Tirinzoni,
Ahmed Touati,
Yingchen Xu,
Anssi Kanervisto,
Scott Niekum,
Amy Zhang,
Alessandro Lazaric
Matteo Pirotta
RLC 2025
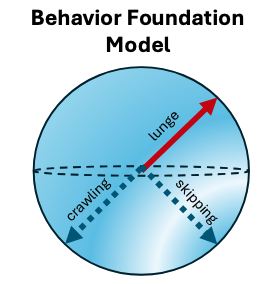
RLZero: Zero-Shot Language to Behaviors without any Supervision
Harshit Sikchi,
Siddhant Agarwal,
Pranaya Jajoo,
Samyak Parajuli,
Max Rudolph,
Peter Stone,
Amy Zhang,
Scott Niekum
In Submission 2025
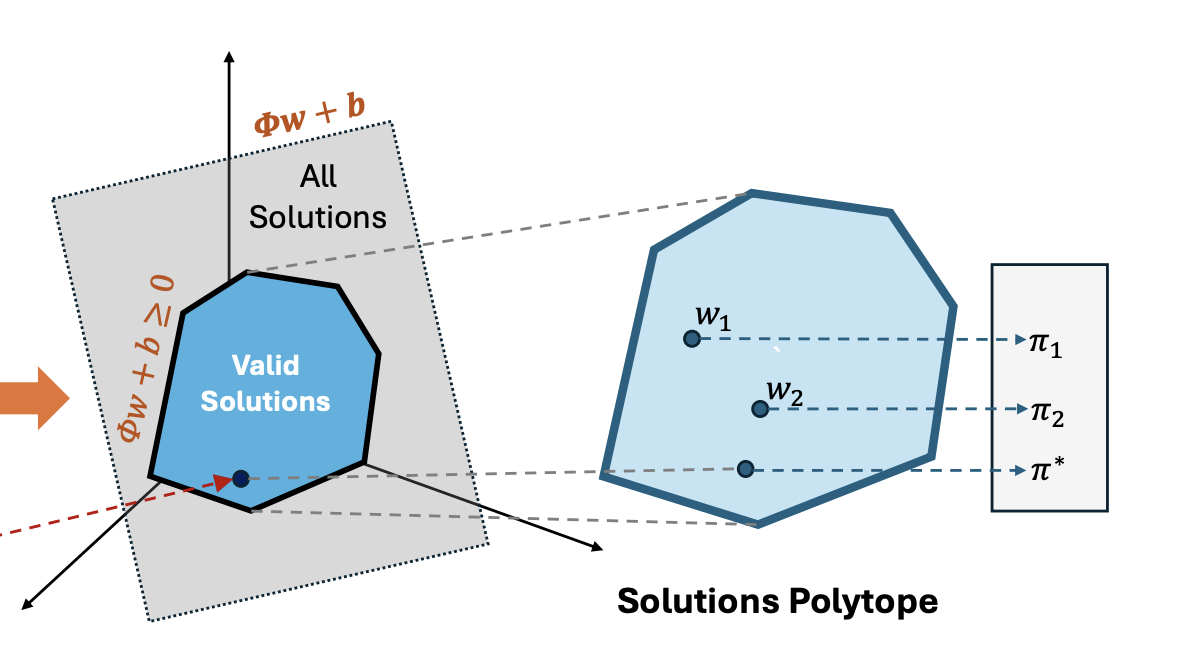
Proto Successor Measure: Representing the Behavior Space of an RL agent
Siddhant Agarwal*,
Harshit Sikchi*,
Amy Zhang,
Scott Niekum, (* Equal Contribution)
ICML 2025
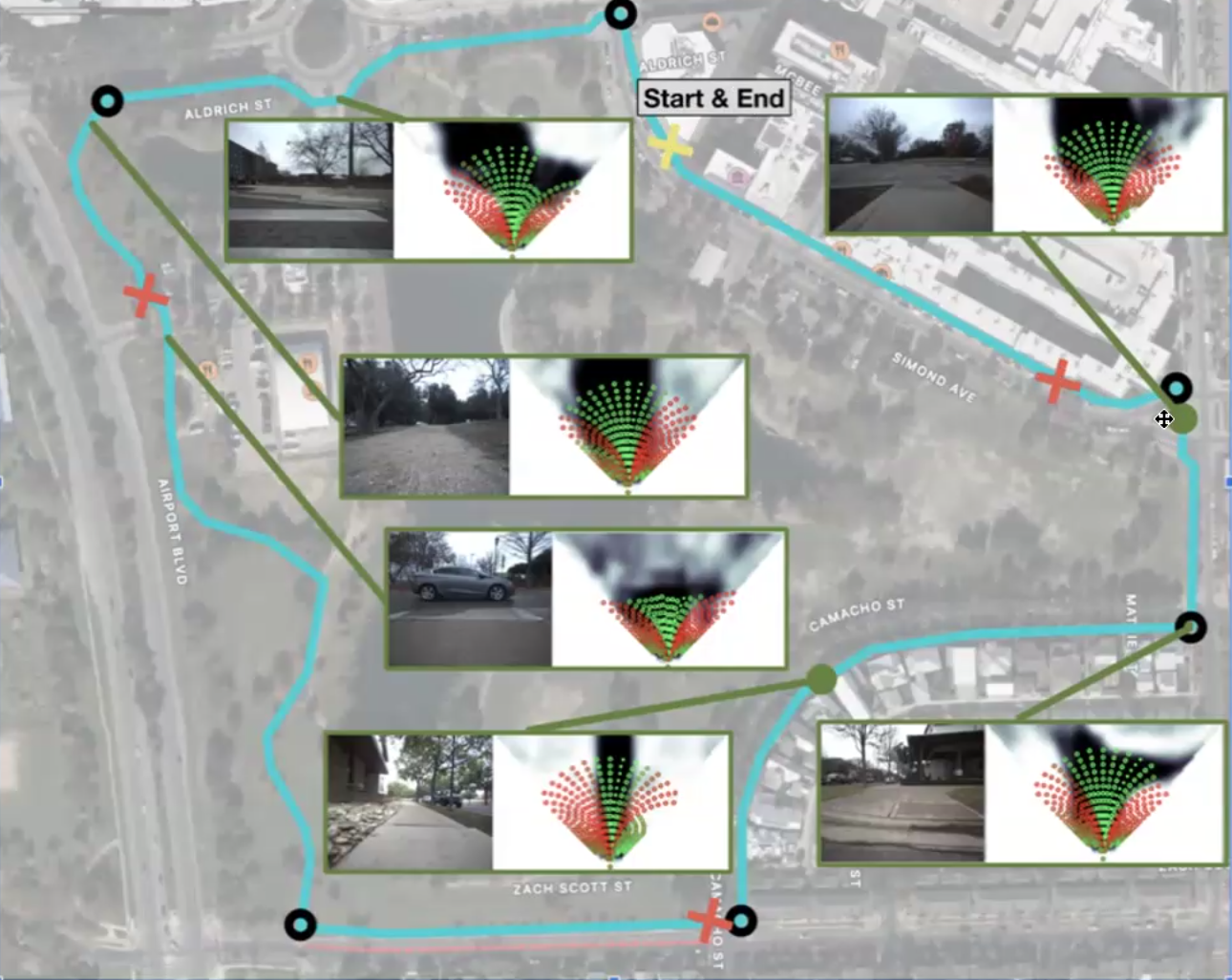
CRESTE: Scalable Mapless Navigation with
Internet Scale Priors and Counterfactual Guidance
Arthur Zhang,
Harshit Sikchi,
Amy Zhang,
Joydeep Biswas
RSS 2025
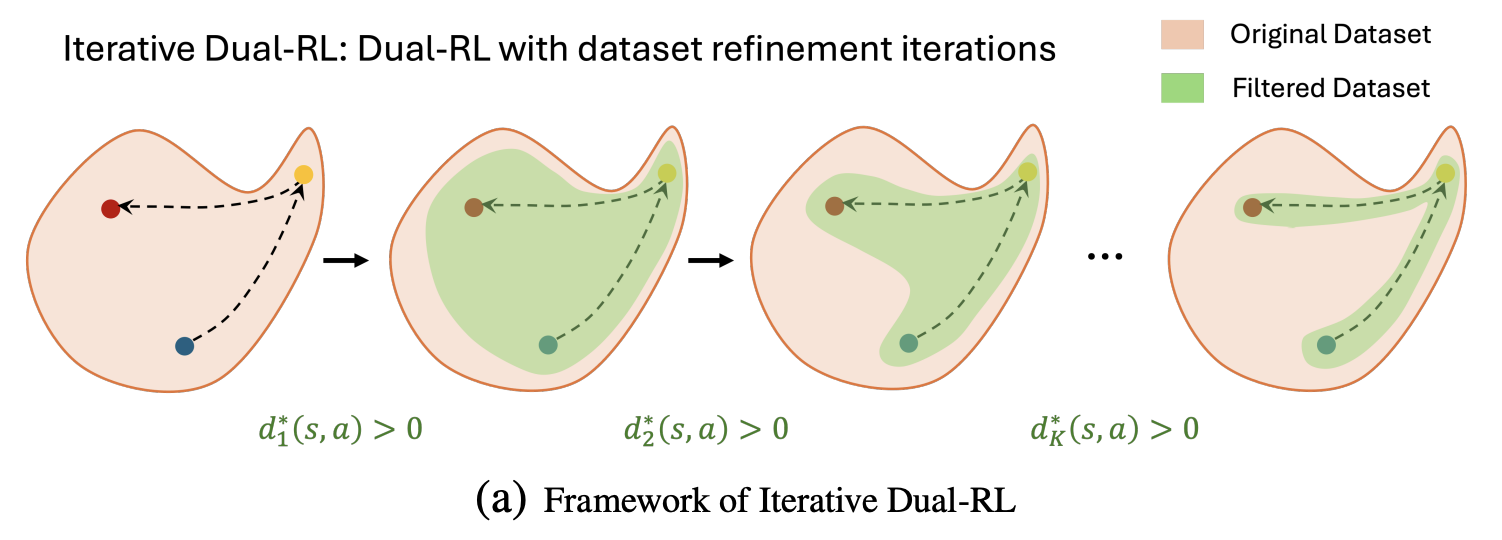
Iterative Dual-RL: An Optimal Discriminator Weighted Imitation Perspective for Reinforcement Learning
Haoran Xu,
Shuozhe Li,
Harshit Sikchi*,
Amy Zhang,
Scott Niekum
ICLR 2025

Scaling Laws for Reward Model Overoptimization in Direct Alignment Algorithms
Rafael Rafailov*,
Yaswanth Chittepu*,
Ryan Park*,
Harshit Sikchi*,
Joey Hejna*,
W. Bradley Knox,
Chelsea Finn,
Scott Niekum, (* Equal Contribution)
NeurIPS 2024

A Dual Approach to Imitation Learning from Observations with Offline Datasets
Harshit Sikchi,
Caleb Chuck,
Amy Zhang,
Scott Niekum,
CoRL 2024
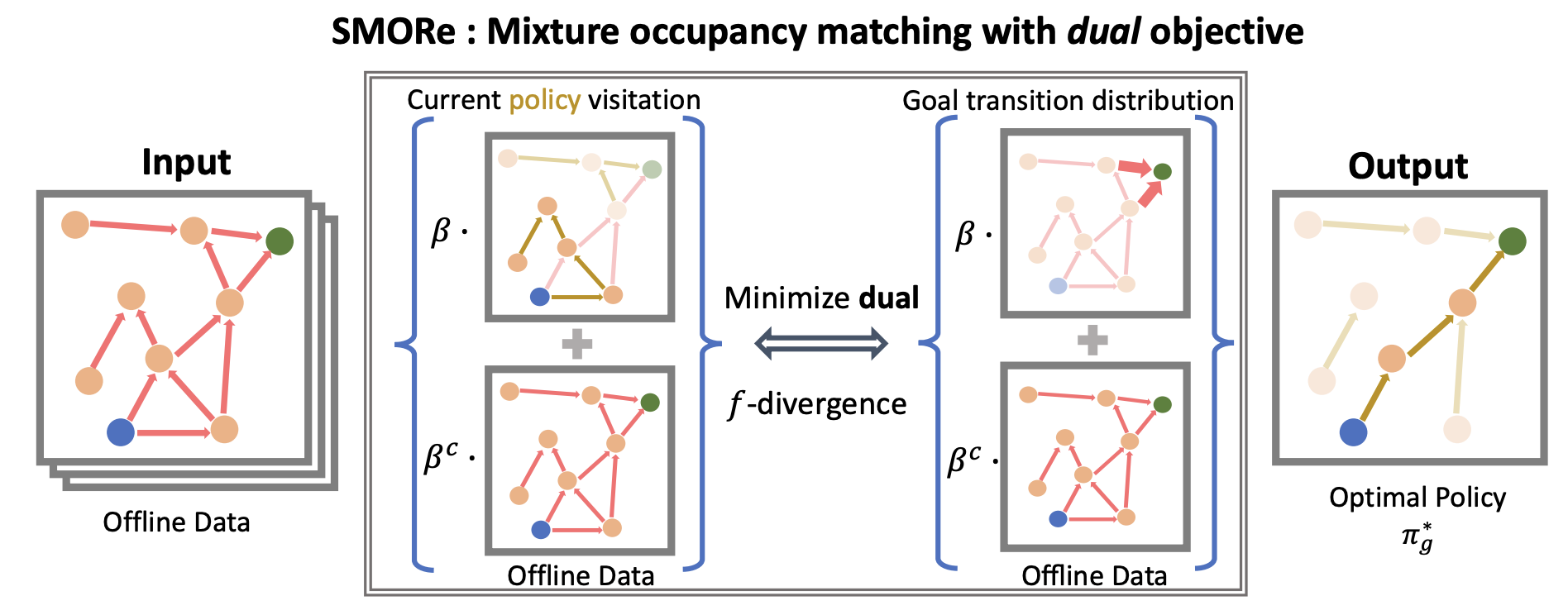
Score Models for Offline Goal Conditioned Reinforcement Learning
Harshit Sikchi,
Rohan Chitnis,
Ahmed Touati,
Alborz Geramifard,
Amy Zhang,
Scott Niekum,
ICLR 2024; NeurIPS GCRL 2023
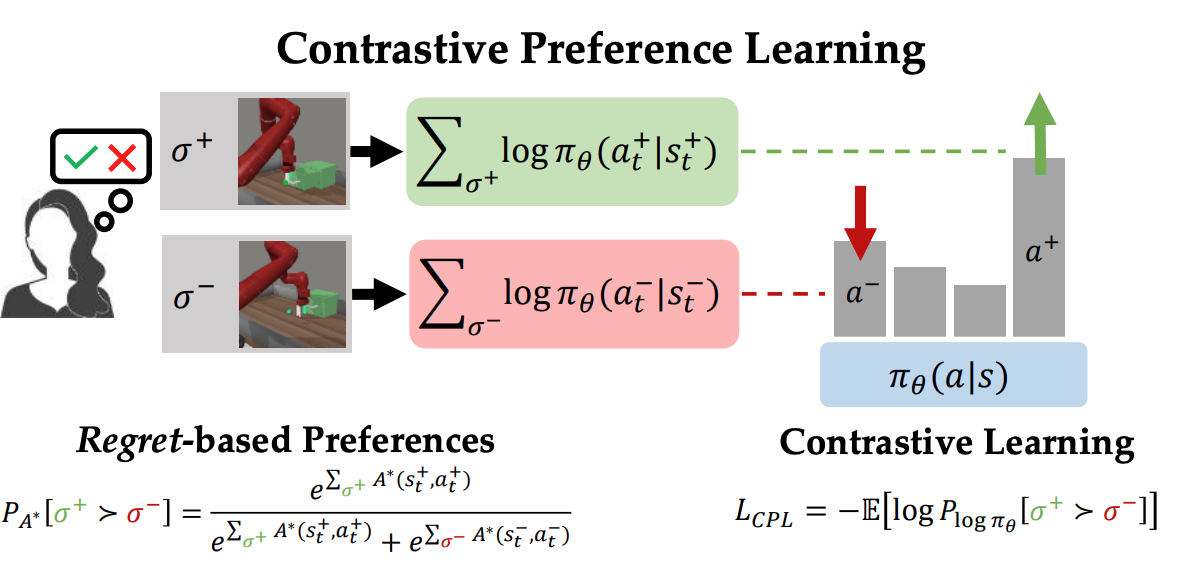
Contrastive Prefence Learning: Learning from Human Feedback without RL
Joey Hejna,
Rafael Rafailov,
Harshit Sikchi,
Chelsea Finn,
Scott Niekum,
W. Bradley Knox,
Dorsa Sadigh
ICLR 2024
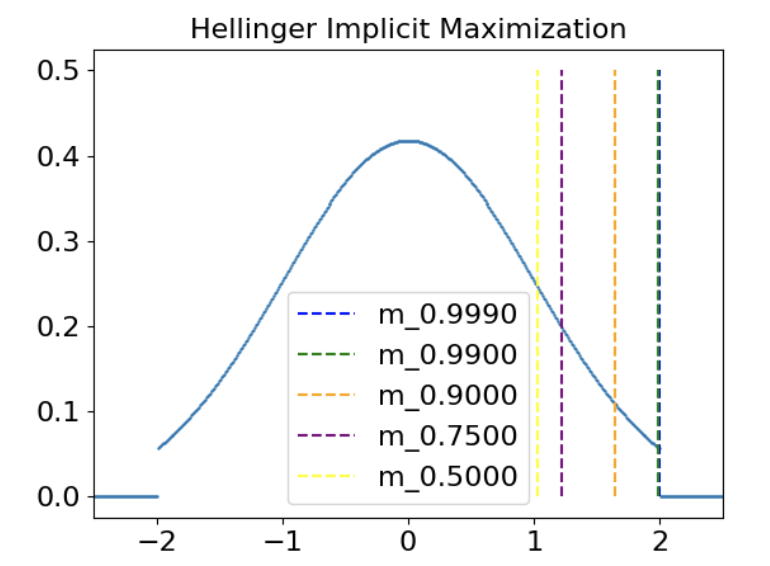
Dual RL: Unification and New Methods for Reinforcement and Imitation Learning
Joey Hejna,
Rafael Rafailov,
Harshit Sikchi,
Qinqing Zheng,
Amy Zhang,
Scott Niekum
ICLR 2024
(Spotlight, Top 5%)
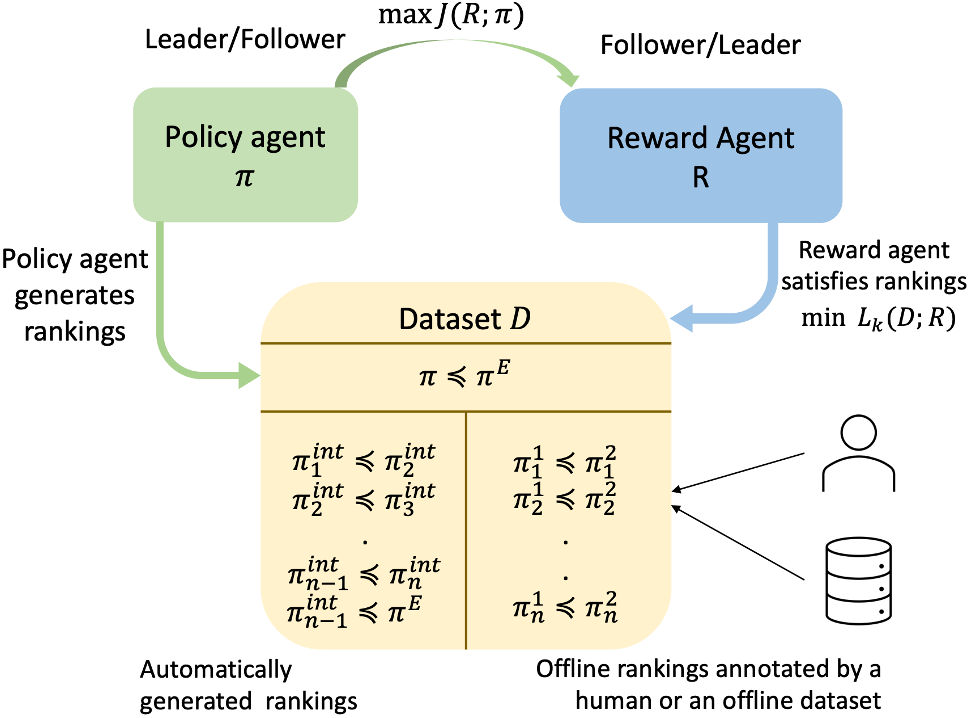
A Ranking Game for Imitation Learning
Harshit Sikchi,
Akanksha Saran,
Wonjoon Goo,
Scott Niekum
TMLR 2022
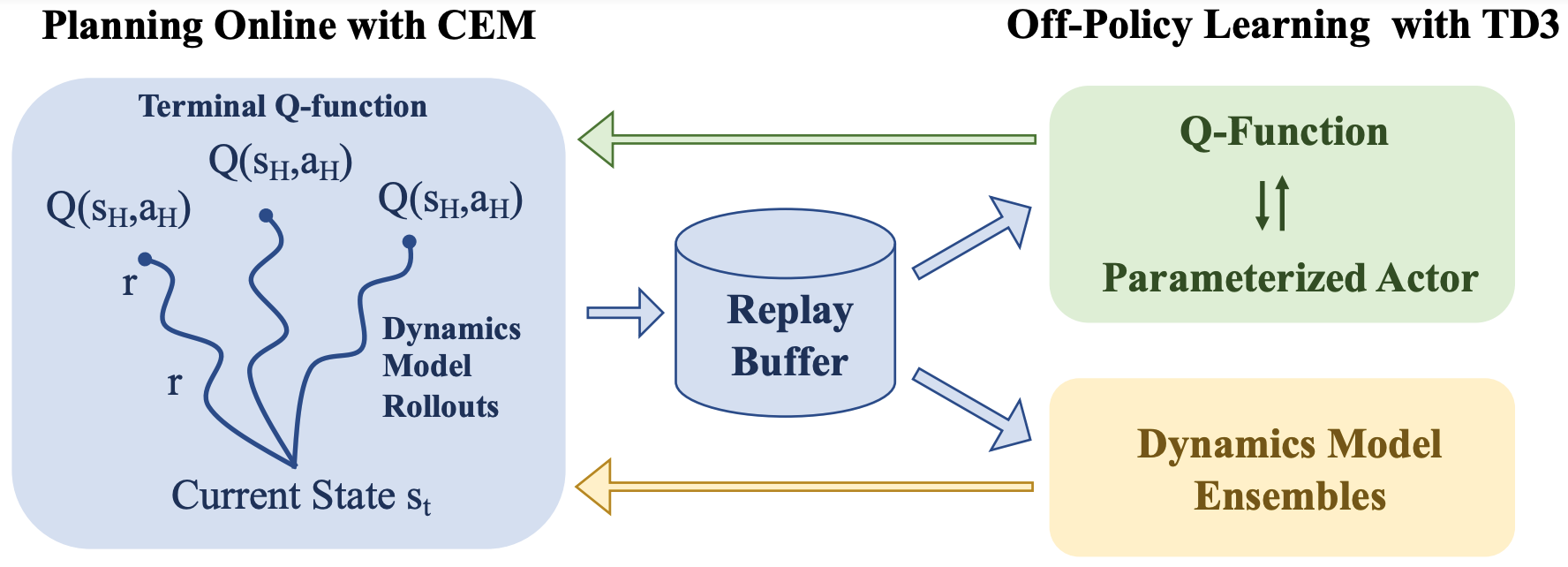
Learning Off-Policy with Online Planning
Harshit Sikchi,
Wenxuan Zhou,
David Held
CoRL 2021
(Best Paper Finalist)
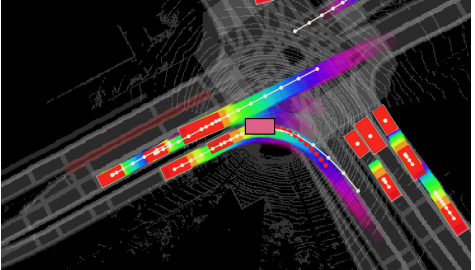
Imitative Planning using Conditional Normalizing Flow
Shubhankar Agarwal,Harshit Sikchi, Cole Gulino, Eric Wilkinson,
IROS BADUE 2022
(Best Paper)
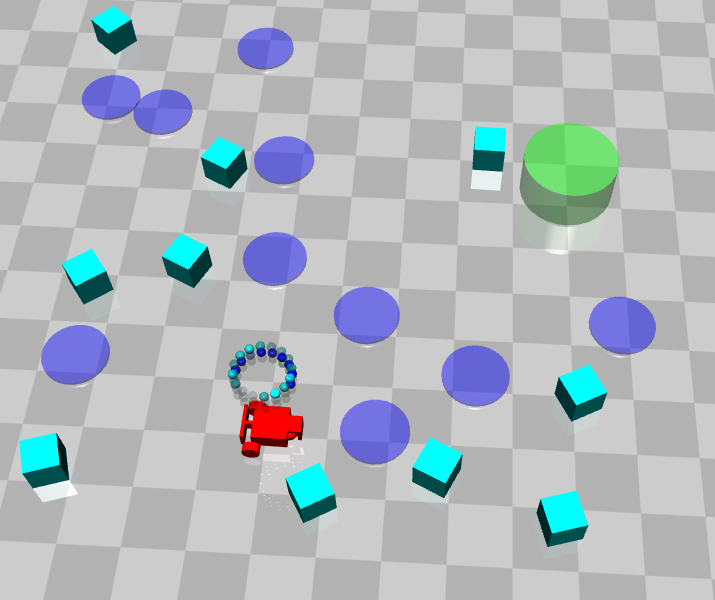
Lyapunov Barrier Policy Optimization
Harshit Sikchi,
Wenxuan Zhou,
David Held
NeurIPS Deep RL Workshop 2020; NeurIPS Real World RL Workshop 2020
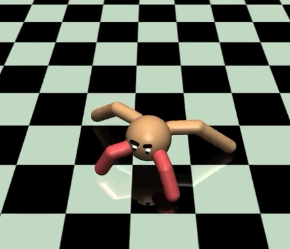
f-IRL: Inverse Reinforcement Learning via State Marginal Matching
Tianwei Ni*,
Harshit Sikchi*,
Yufei Wang*,
Tejus Gupta*
Ben Eysenbach
Lisa Lee (* Equal Contribution - Dice Rolling)
NeurIPS Deep RL Workshop 2020; NeurIPS Real World RL Workshop 2020
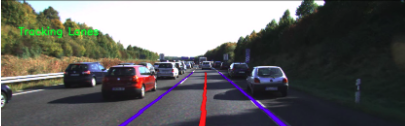
Robust Lane Detection Using Multiple Features
Tejus Gupta*,
Harshit Sikchi*,
Debashish Chakravarty (* Equal Contribution - Dice Rolling)
Intelligent Vehicle(IV) 2018